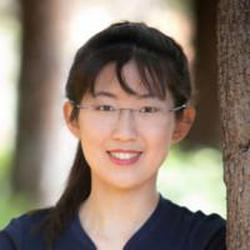
Reinforcement Learning for Knowledge Graph Reasoning
A Talk by Victoria Lin (Senior Research Scientist, Salesforce Research)
About this Talk
Large-scale knowledge graphs (KGs) support a variety of downstream NLP applications such as semantic search and chatbots.
Answering arbitrary user questions over KGs often requires reasoning over multiple inter-related facts.
In a common setup where the topic entity and the target relation are known, the problem can be formulated as a partially observable Markov decision process (POMDP), where a policy-based agent sequentially extends its inference path from the topic entity until it reaches a target.
However, in an incomplete KG environment, the agent receives low-quality rewards corrupted by false negatives in the training data, which harms generalization at test time. Furthermore, since no golden action sequence is used for training, the agent can be misled by spurious search trajectories that incidentally lead to the correct answer.
In this work, we propose two modeling advances to address both issues: (1) We reduce the impact of false negative supervision by adopting a pretrained one-hop embedding model to estimate the reward of unobserved facts; (2) We counter the sensitivity to spurious paths of on-policy RL by forcing the agent to explore a diverse set of paths using randomly generated edge masks.
Our approach significantly improves over strong path-based KGQA baselines and is comparable or better than embedding-based models on several benchmark datasets.