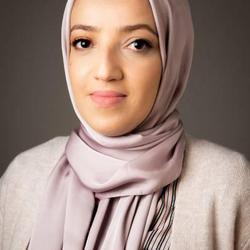
Leveraging the Semantics of Adverse Childhood Experiences for Explainable AI Recommendations
A Talk by Nariman Ammar (Research Fellow, University of Tennessee Health Science Center - ORNL's Center for Biomedical Informatics)
About this Talk
Classic ML approaches to AI cannot provide a full scientific understanding of the inner workings of the underlying models.
This raises credibility issues due to the lack of transparency and generalizability.
Explainable AI is an emerging approach for promoting credibility in mission-critical areas (e.g., Medicine) by combining ML with explanatory techniques that explicitly show what the decision criteria are and why (or how) they have been established.
One way to achieve this is by considering formal ontologies as an integral part of the learning process.
A knowledge graph can serve as a contextually aware dynamic backend that stores data in a certain domain as entities and relationships using a graph model, which abides by an ontology.
AI applications (e.g., recommendation systems and chatbots) that are based on knowledge graphs are easier to train with minimal maintenance.
In this talk, I will demonstrate a knowledge-driven, evidence-based recommendation system and chatbot that utilize evidence collected from literature, a population health observatory , and “common sense” knowledge along with semantic inference of causal epidemiological relations to build a personalized Health KG, and uses that graph to automate screening for social needs and navigate those in need to available resources.
Technologies used: Protege (RDFS/OWL for Ontology development), Neo4j (GraphDB), Neosemantics (RDFS to Property Graph converter), Google DialogFlow (Chatbot engine).
This talk is based on a recent Journal article published in JMIR Medical Informatics