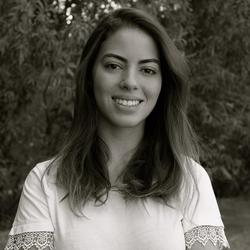
GraphEDM: A Unified Framework for Machine Learning on Graphs
A Talk by Ines Chami (Researcher, Stanford University, Institute for Computational & Mathematical Engineering)
About this Talk
There has been a surge of recent interest in learning representations for graph-structured data.
Graph representation learning methods have generally fallen into three main categories, based on the availability of labeled data.
The first, network embedding (such as shallow graph embedding or graph auto-encoders), focuses on learning unsupervised representations of relational structure.
The second, graph regularized neural networks, leverages graphs to augment neural network losses with a regularization objective for semi-supervised learning.
The third, graph neural networks, aims to learn differentiable functions over discrete topologies with arbitrary structure.
In this talk, we present our recent GraphEDM unified framework which bridges the gap between these disparate bodies.
We use GraphEDM to describe many popular graph representation learning methods such as DeepWalk and Graph Convolutional Neural Networks.