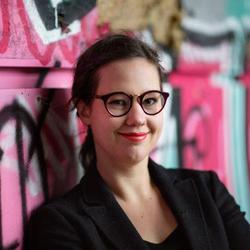
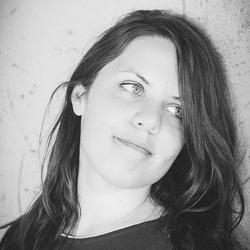
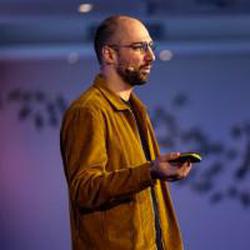
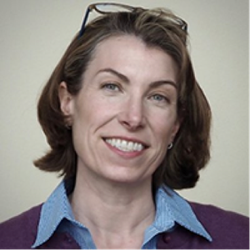
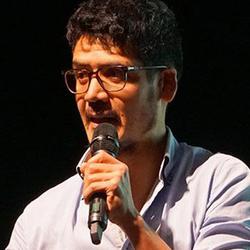
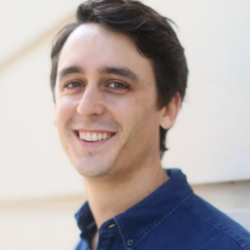
AI + Knowledge - a match made in heaven?
A Talk by Nathan Benaich , Isabelle Augenstein , Giuseppe Futia , Fabio Petroni , Katariina Kari and Amy Hodler
This Talk Has Finished
This talk was only available to attend live. A replay is not being made available.
About this Talk
What does graph have to do with machine learning?
A lot, actually. And it goes both ways
Machine learning can help bootstrap and populate knowledge graphs.
The information contained in graphs can boost the efficiency of machine learning approaches.
Machine learning, and its deep learning subdomain, make a great match for graphs. Machine learning on graphs is still a nascent technology, but one which is full of promise.
Amazon, Alibaba, Apple, Facebook and Twitter are just some of the organizations using this in production, and advancing the state of the art.
More than 25% of the research published in top AI conferences is graph-related.
Domain knowledge can effectively help a deep learning system bootstrap its knowledge, by encoding primitives instead of forcing the model to learn these from scratch.
Machine learning can effectively help the semantic modeling process needed to construct knowledge graphs, and consequently populate them with information.
Kindly sponsored by:
👉 Book Your Workshop Ticket Now, get Free Conference Ticket, catch 30+ Top Speakers!
Key Topics
- What can knowledge-based technologies do for Deep Learning?
- What is Graph AI, how does it work, what can it do?
- What's next? What are the roadblocks and opportunities?
Target Audience
- Machine Learning Practitioners
- Data Scientists
- Data Modelers
- CxOs
- Investors
Goals
- Explore the interplay between machine learning and knowledge based technologies
- Answer questions that matter
- How can those approaches complement one another, and what would that unlock?
- What is the current state of the art, how and where is it used in the wild?
- What are the next milestones / roadblocks?
- Where are the opportunities for investment?
Session outline
- Introduction
- Meet and Greet
- Setting the stage
- Knowledge Graphs, meet Machine Learning
- How can machine learning help create and populate knowledge graphs?
- What kind of problems can we solve by using it?
- Where is this used in production?
- What is the current state of the art in knowledge graph bootstrapping and population?
- What are the major roadblocks / goals, how could we address them, and what would that enable?
- Who are some key players to keep an eye on?
- Graph Machine Learning
- What is special about Graph Machine Learning?
- What kind of problems can we solve by using it?
- Where is it used in production?
- What is the current state of the art?
- What are the major roadblocks / goals, how could we address them, and what would that enable?
- Who are some key players to keep an eye on?
Format
- Extended panel
- Expert discussion, coordinated by moderator
- 2 hours running time
- Running time includes modules of expert discussion, interspersed with modules of audience Q&A / interaction
Level
- Intermediate - Advanced
Prerequisite Knowledge
- Basic understanding of Knowledge Graphs
- Basic understanding of Machine Learning / Deep Learning